Ahead of the upcoming launch of our next-generation AI Agent to support our users with content discovery and coaching, I thought it right to open up a dialogue on an important topic: how to measure the success of an AI agent rollout within a learning management system (LMS) setting.
Implementing AI agents paints a dream of being able to transform the way we learn, but what constitutes effective use of the technology? Below, I have articulated some of the key metrics and strategies that we plan to adopt at 5app to do just that.
1. User engagement metrics
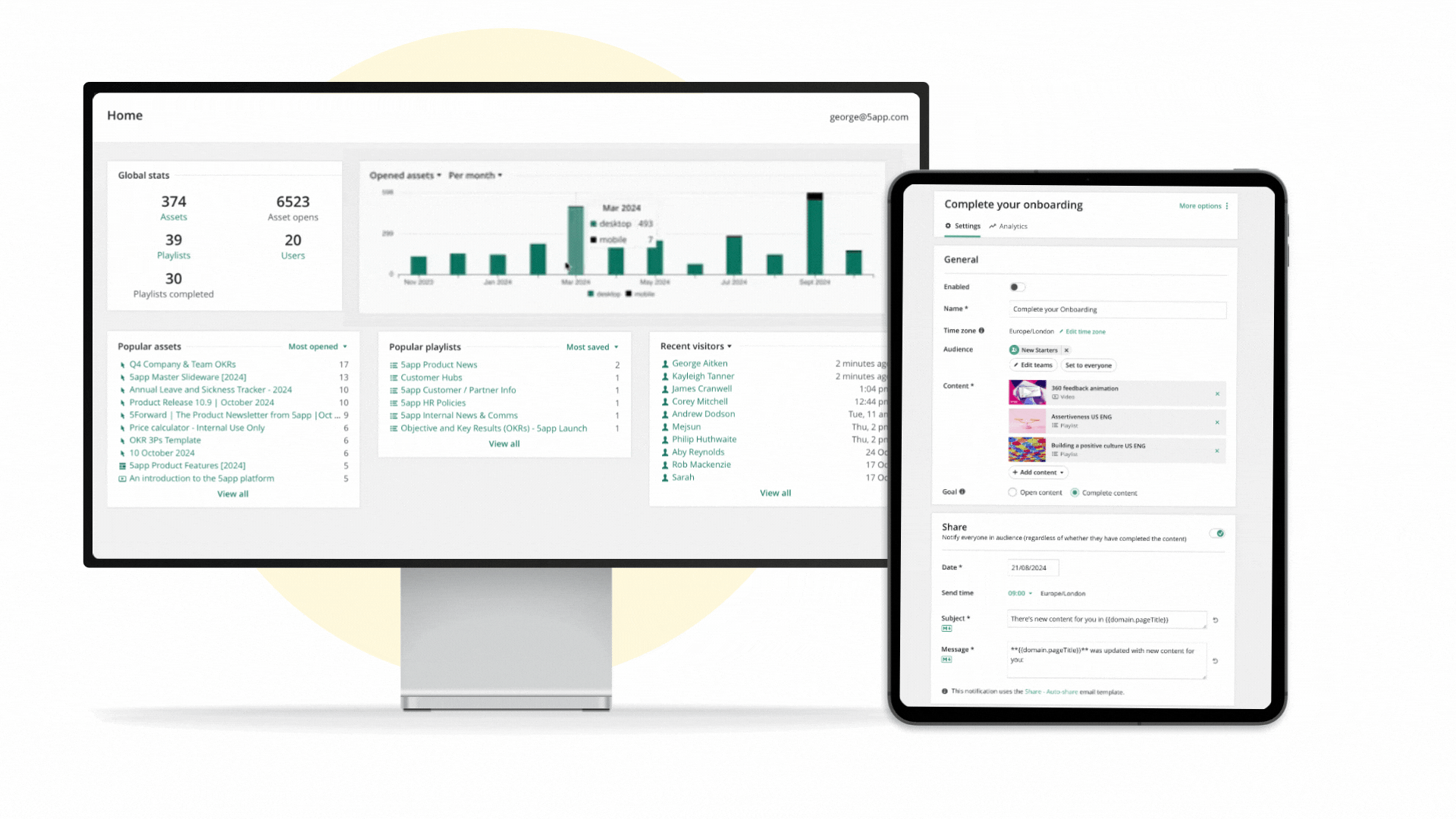
Straight out of the gate, let's talk about learner engagement. The interaction rates of users with the AI agent is a strong barometer for engagement levels. This includes tracking how many questions are being asked, commands given or topics explored. High interaction rates suggest that users are finding the AI agent useful and engaging.
Another indicator is session duration. Are users spending a significant amount of time interacting with the AI? Longer sessions typically suggest that they’re finding value in those interactions.
2. User adoption rates
By extension of this, user adoption is another key area we're considering. How quickly are users embracing the AI agent after its rollout? High adoption rates within a short time frame suggest that users are open to exploring its benefit, but the primary metric to track here should be repeat usage. If users keep coming back to the AI agent, that’s a solid indicator that it’s meeting their needs.
3. User satisfaction and feedback
Next, we need to hear from our users directly. Elevating their experience is part of our company vision here at 5app. Surveys and feedback forms can work well in this scenario. After interacting with the AI agent, we plan to ask users about their experience. Was it easy to use? Did they get the information they needed?
These user insights can guide us in tweaking the configuration of the agent, with everything from personality, to how responses are structured to ensure satisfaction is maximised.
Another measure we plan to adopt is to ask how likely users are to recommend the AI agent to their colleagues, on a simple 1-10 scale (either in-platform or via email), This will allow us to calculate a Net Promoter Score (NPS) at regular intervals to obtain a 'pulse check' of overall satisfaction.
4. Adaptability and learning
An AI agent's ability to learn and improve over time is also a major indicator of success. We plan to track not only how responses evolve based on user interactions, but also the accuracy of responses. This continuous improvement helps ensure that the AI remains relevant and helpful.
5. Operational efficiency
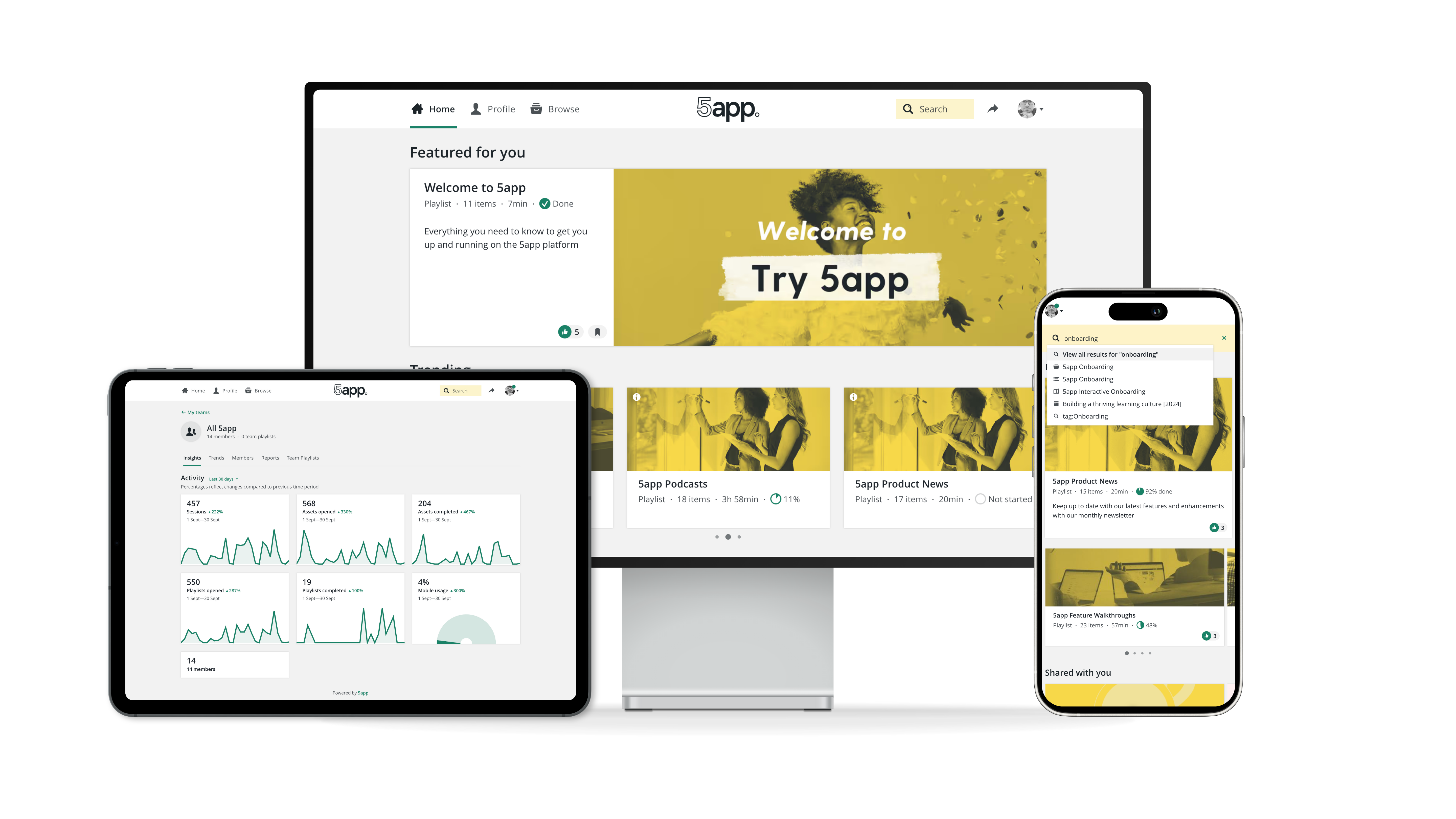
Operational efficiency is another critical measure of success. Time saving is a pragmatic performance indicator in this regard. Are users spending less time searching for information because they have the AI agent at their disposal, versus before the technology was available? If yes - it’s likely the adoption of the technology is helping.
6. Learning outcomes
At the end of the day, our goal is effective learning. To assess whether the AI agent contributes to meaningful knowledge retention, we hope to implement knowledge retention tests. By measuring users’ understanding before and after interacting with the AI agent, it is possible to get a clearer picture of its impact. This, however, is perhaps much harder to complete and manage when you have hundreds of thousands of users, and we certainly don't wish to add additional burden to learners. Therefore, much like exit polls in a political scenario, we plan to identify a representative sample of users to roll out an assessment of this nature, certainly during the initial pilot phase.
Measuring the success of your AI agent rollout
In conclusion, measuring the success of an AI agent rollout in an LMS requires a multifaceted approach. It’s about looking at the entire user experience and not only monitoring platform usage, but also being proactive and engaging in a dialogue with the users themselves.
At 5app, we’re committed to using these insights to refine our AI tools and make sure they provide real value to our users. By focusing on these types of metrics, we can continue to enhance the learning experience and help our users achieve their goals.